Marion Oswald
Chapter 5 of the Centre for Justice Innovation’s report, Just Technology, focuses upon the use of machine learning algorithms to categorise or make predictions, and the subsequent use of those predictions within criminal justice decision-making. This, in my view, is an area that deserves considerable attention, but one where the devil, as always, is in the detail.
The public polling in the report indicates some level of support for algorithms to supplement or assist human decisions. There appears to be almost no support, however, for algorithms to have a decision-making role. Those I’ve spoken to in the police are clear that they would not want this either. Despite this, the introduction of an algorithm into a decision-making process – often including an element of discretion – has the potential change or disrupt that process, in positive or negative ways.
The Just Technology report recognises this and recommends the shadowing of algorithmic applications in key justice decisions ‘to ascertain whether they more accurately predict better outcomes than human decision makers’ (Recommendation 13). I certainly agree that finding robust ways of 'testing' unproven technology within the criminal justice system is of crucial importance (and my model called 'experimental proportionality' aims to contribute to addressing this need). It will be vital however to assess each process in context before drawing conclusions as to whether an algorithm is more ‘accurate’ or makes ‘better’ decisions. What does more ‘accurate’ and ‘better’ mean when assessing the impact of algorithms?
The problem with many claims for algorithmic accuracy (versus human judgements) is that they claim far more for the algorithmic output than it really is. An algorithmic output is a probability that the individual in question has a certain similarity – in terms of their personal characteristics, criminal record and so on – to people in the past. That’s all. It’s not a determination of risk, or of anything else, other than the narrow categorisation or probability for which the algorithm was designed.
So we must take care when comparing algorithmic outputs to human decisions and deciding which is ‘better’. We first need to understand the decision that is to be taken, the factors that feed into it (including the algorithmically generated one), what element of discretion it contains and the process overall. We mustn’t fall into the trap of assuming that the forecast or classification is the only way of assessing the ‘rightness’ or ‘wrongness’ of the overall decision. As I discuss in a forthcoming article (preprint here), making this assumption may inadvertently change the question that the public sector decision-maker has to answer - questions that often involve tricky concepts such as reasonableness or necessity, or assessments of risk linked with the offender’s family or personal circumstances. The offender was predicted to have a medium risk of re-offending if released on bail, but she was released by the police force anyway, and she has re-offended, and so the human decision was wrong. But is that the correct conclusion? The officer could – and should - have considered other relevant factors, such as family and social connections, and any plan to address the offender’s personal circumstances (not easy to ‘datafy’). Policy factors might have been in play too. A poor outcome in the future does not necessarily mean that the human decision at the time was the wrong one.
One final comment: it’s often said that there’s no law governing this whole area, or if there is law, then it’s no good for this new tech. I disagree. In my recent research, I’ve looked at a number of long-standing English administrative law rules designed to regulate the discretionary power of the state. Algorithms do not exist in a vacuum; they are deployed within decision-making processes involving humans. And where the exercise of state power and discretion is concerned, administrative law has a role. I like to think of it as old law for new algorithmic tricks. I argue that the duty to give reasons, the rules around relevant and irrelevant considerations, and fettering discretion can signpost key principles for the deployment of algorithms within public sector settings.
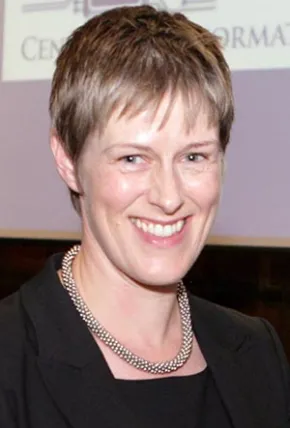
Marion Oswald is the founder and director of the Centre for Information Rights and a Senior Fellow in Law at the University of Winchester. She is a solicitor (non-practising), with previous experience in legal management roles within private practice, international companies and UK central government. She has worked extensively in the fields of data protection, freedom of information and information technology, having advised on a number of information technology implementations, data sharing projects and statutory reforms. She publishes and speaks on the interaction between law and digital technology and has a particular interest in the use of information and innovative technology by the public sector. She is a member of the National Statistician’s Data Ethics Advisory Committee and a member of the Royal Society Working Group on Privacy Enhancing Technologies.